When John Donahoe, ServiceNow’s new CEO, delivered his opening keynote at Knowledge17, he announced the Intelligent Automation Engine — the company’s new machine learning layer that sits on top of the its automation platform – and explained why he believes that machine learning will be a critical part of every enterprise’s future.
Donahoe’s announcement was yet another signal that machine learning is entering the mainstream. ServiceNow’s commitment to the machine learning domain communicated to its customers that machine learning is something that will be an essential part of their future, and which they must take seriously. While it will be applied across the spectrum of the ServiceNow platform, it was clear that IT Operations is a major focus of its application.
Based on the reaction at the event, however, it was apparent that it was the first time that many people in the audience had seriously contemplated the impact and benefit that machine learning could provide within the IT Operations space. With ServiceNow’s commitment to machine learning, it will now be top-of-mind for all IT Operations leaders — and lead them to explore more advanced applications of this breakthrough technology.
An Integrated IT Operations Future
ServiceNow’s approach to machine learning is a great starting point for enterprises attempting to leverage this technology to improve operations. Its approach is to use basic machine learning algorithms to automatically predict and prevent outages, to automatically categorize and route workflows, and to predict operational performance.
But those uses of machine learning are the starting point, not the end. In fact, one of the greatest uses of this new technology is to close one of the largest gaps in IT Operations: the persistent divide between the various operational functions.
Most IT organizations manage event management, incident management, analytics and monitoring independently — and typically in isolation by different functional teams. Why?
While there are many reasons for this divide, organizations have had difficulty overcoming it because it has been too challenging to make all the pieces fit together in a way that didn’t impact any given team’s ability to operate effectively. So, everyone just did their own thing.
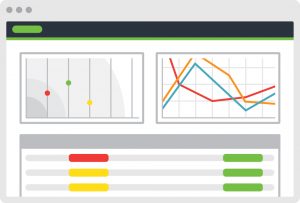
Several years ago, ServiceNow partner, Evanios, realized that it could apply machine learning techniques to the IT Operations space to close this gap.
Its solution fully integrates event management, automated incident resolution, predictive analytics and monitoring into a unified workflow running within the ServiceNow environment — enabling the organization to work together seamlessly with consistent and predictive information. The result is a more reliable and resilient IT operating platform — and it’s made possible by the company’s application of machine learning strategies.
The Promise of Machine Learning in IT Operations
Evanios’ dynamic and highly integrated approach works by collecting and aggregating a large and comprehensive set of operational data and then using advanced algorithms to act on the resulting insights.
For instance, Evanios assimilates event, discovery, and service request data to identify new technology assets, and automatically provision monitoring of those assets using pre-set policies and rules.
This automation solves one of the greatest gaps in IT today: keeping monitoring profiles up-to-date and ensuring that the IT organization is consistently monitoring critical assets from the point of inception.
As another example, the company uses machine learning to provide IT operations teams with a broad range of proactive and predictive capabilities, such as the ability to score events by business impact, automate root cause analyses, provide real-time impact severity predictions and even compare predicted versus actual business impact both in real time and retrospectively.
It’s the company’s ability to integrate and correlate a diverse and rich data set and then apply advanced machine learning algorithms to that data that makes all of these capabilities possible, and enables IT Operations to function more effectively.
Therein lies the real promise of machine learning: its ability to augment and enhance the IT operations team’s ability to execute.
The combination of rich data sets and the practical application of artificial intelligence, such as machine learning, will reshape the fundamental IT operating paradigm.
ServiceNow’s new capabilities and the emphasis that they are placing on its use within IT operations will help operations teams understand that this is their new reality. But the most progressive organizations will quickly realize that they must apply these techniques in much more comprehensive and advanced ways to thrive in this new era of machine learning and automation.
The Intellyx Take
There are three trends that IT Operations cannot escape: the growing complexity of the enterprise technology stack, the increased demand to innovate and leverage technology to drive competitive advantage and the never-ending pressure to continually manage all of this complexity and meet this increased demand with fewer and fewer resources.
In many ways, simultaneously responding to these types of competing pressures is part of the digital transformation story. IT organizations — and specifically IT Operations teams — must adopt and adapt new technologies and strategies to deal with them effectively.
When applied intelligently, advanced machine learning techniques will enable the IT Operations team to rise to the challenges of the digital era. Those organizations that ignore these advances, believing that they can survive without changing or adapting their management approaches and supporting technologies, will find themselves fighting a losing battle.
But for those IT Operations teams that understand the power of applying advanced machine learning approaches and which aggressively embrace and adopt them to reshape their IT operations model, the future is bright indeed.
Copyright © Intellyx LLC. Evanios and ServiceNow are Intellyx clients. Intellyx retains full editorial control over the content of this paper. Image credit: Evanios.
Comments