“On two occasions I have been asked, ‘Pray, Mr. Babbage, if you put into the machine wrong figures, will the right answers come out?’” wrote computing pioneer Charles Babbage in 1864. “I am not able rightly to apprehend the kind of confusion of ideas that could provoke such a question.”
And thus, the fundamental software principle of ‘garbage in, garbage out’ was born. Today, however, artificial intelligence (AI) has raised the stakes on Babbage’s conundrum, as the ‘garbage out’ from AI leads to appalling examples of bias.
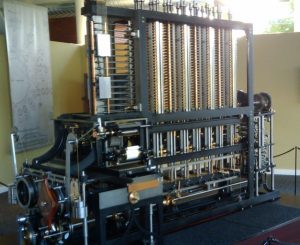
AI – in particular, both machine learning and deep learning – take large data sets as input, distill the essential lessons from those data, and deliver conclusions based on them.
For example, if you want to use AI to make recommendations on who best to hire, feed the algorithm data about successful candidates in the past, and it will compare those to current candidates and spit out its recommendations.
Just one problem. If the input data are biased – say, consisting of mostly young white males (our ‘garbage in,’ as it were), then who will the AI recommend? You guessed it: mostly young white males (predictably, the ‘garbage out’).
As Babbage would likely affirm, the fault here is with the input data, not the AI algorithms themselves. However, there is more to bias than bad data. “The data itself is just, well, data,” says Max Pagels, machine learning partner at Fourkind. “It’s not socially biased, it’s just a bunch of numbers. A dataset needs to be constructed carefully to avoid inducing social bias, but it’s not biased in and of itself.”
Whether the AI algorithms are themselves biased is also an open question. “[Machine-learning algorithms] haven’t been optimized for any definition of fairness,” says Deirdre Mulligan, associate professor, UC Berkeley School of Information. “They have been optimized to do a task.”
Optimizing the AI algorithm and constructing data sets to avoid bias, however, go hand in hand. “Imagine a self-driving car that doesn’t recognize when it ‘sees’ black people,” warns Timnit Gebru, PhD, from the Fairness, Accountability, Transparency, and Ethics (FATE) group at the Microsoft Research New York lab. “That could have dire consequences.”
She points out that data may not be the only problem. “[Deep learning-based decision-making algorithms] are things we haven’t even thought about, because we are just starting to uncover biases in the most rudimentary algorithms,” Gebru adds.
Unsurprisingly, facial recognition software that improperly or inadequately recognizes people of color is a lightning rod for controversy. “If the training sets aren’t really that diverse, any face that deviates too much from the established norm will be harder to detect, which is what was happening to me,” explains Joy Buolamwini, graduate researcher at MIT Media Lab and founder of Algorithmic Justice League and Code4Rights. “Training sets don’t just materialize out of nowhere. We actually can create them. So there’s an opportunity to create full-spectrum training sets that reflect a richer portrait of humanity.”
Read the entire article at https://www.forbes.com/sites/jasonbloomberg/2018/08/13/bias-is-ais-achilles-heel-heres-how-to-fix-it/.
Intellyx publishes the Agile Digital Transformation Roadmap poster, advises companies on their digital transformation initiatives, and helps vendors communicate their agility stories. As of the time of writing, IBM and Microsoft are Intellyx customers. None of the other organizations mentioned in this article are Intellyx customers. Image credit: Nick Richards.