BrainBlog for Krista Software by Jason Bloomberg
Part 4 of the Intellyx Intelligent Automation Series
In a recent episode of Star Trek: Discovery, the crew struggled with the question of how to trust their newly sentient ship’s computer Zora.
The issue of trust came to a head when Zora made a unilateral decision the crew didn’t like. In the face of such insubordination, is there any way the crew could trust Zora to follow the chain of command?
Today’s AI is many years away from suddenly waking up sentient, but the question of trust is front and center in every professional’s mind.
If there’s a chance that some AI-driven software might get an answer wrong – either clearly incorrect or perhaps more perniciously, subtly biased – then how can we ever trust it?
A Different Kind of Software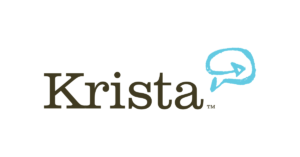
The reason that people struggle with trusting AI is because AI works differently from other software.
AI depends upon both models and data sets. An AI model is a program (or simply an algorithm) that relies on a data set to recognize patterns and make predictions or decisions.
The behavior of this model, therefore, depends upon the data you feed it. Good data yield good results, whereas biased data yield biased results.
The data sets we feed our AI models, however, tend to be quite large and opaque. We typically have no idea what problems such data have, bias or otherwise. You might even say that one of the primary uses of AI is to uncover such issues.
Having a program tell us what’s wrong with our data, however, is a far cry from providing useful insights for our business or recommendations as to what decisions we should make.
How can we trust the results from our AI, therefore, if we can’t trust our data – and we have no way of uncovering their underlying issues other than the AI models themselves?
Putting Humans in the Loop
Trust, of course, must be earned – even when the party in question is AI.
The best way to build trust in an AI routine is gradually over time. Run the routine, have people evaluate the results, and repeat as needed.
Sometimes the results will be off. In such situations, either adjust the model or the data sets to better represent the goals of the initiative. Then rinse and repeat.
Over time, the AI’s results will improve, as AI is able to learn over time. The people using the routine will see this improvement as the AI returns gradually improving answers.
Eventually, those answers will be good enough, where ‘good enough’ depends upon the business goals the organization is looking to achieve with its AI. But not only are the results sufficient, the people using the AI will know that the answers are good enough, because they have seen the AI improve with use.
In other words, this iterative approach to improving AI results builds trust – in both the models and the data sets feeding them.
Read the entire BrainBlog here.